Data skills assessment
Accurate, efficient, objective
Transform your data talent recruitment, save time and cost, hire and upskill better.
Skills-based
Accurate
Efficient
Skills-based
Rely on proven standardized, objective assessments rather than misleading resumes or biased judgements.
People come into data science from a variety of educational backgrounds and professional experiences which make it challenging to use traditional screening and assessment tools and techniques.
It is critical for organizations to adopt skill-based hiring and upskilling to achieve their talent goals and establish high-performing and diverse data talent.
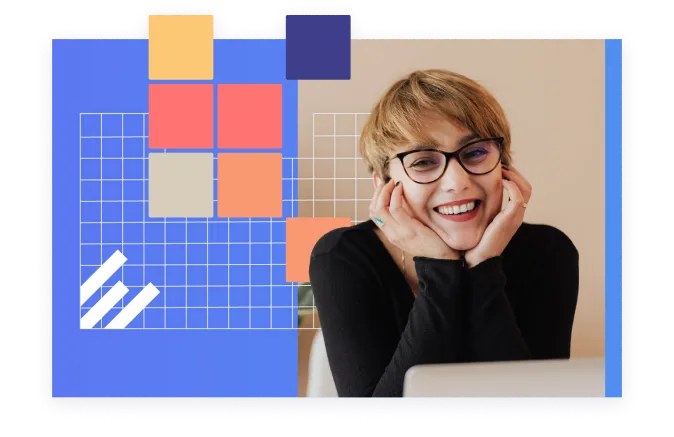
Accurate
Ask the right questions for a variety of skills and roles created by Elements experts.
Our proprietary inventory of questions measure specific skill and knowledge areas accurately. We rely on the skills framework developed by Initiative for Analytics and Data Science Standards (IADSS), an industry best practice in professional standards in data science and data literacy.
Our assessment approach is based on highly granular topics within each area so we can be very targeted on measuring specific skills.
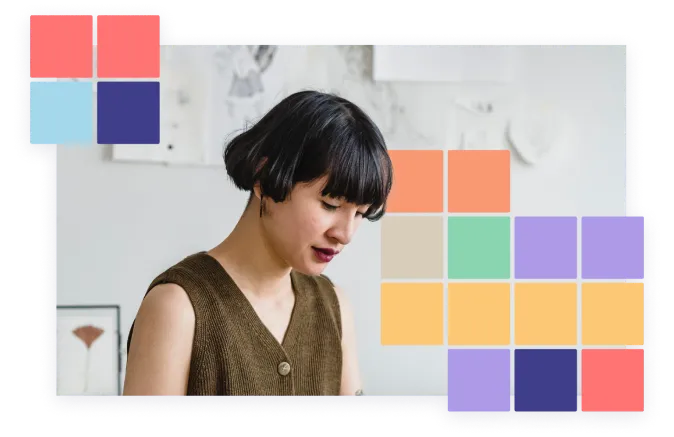
Efficient
Save significant time of the recruitment & training teams, track results, access to analytics; hire faster in competitive talent market, spot the training needs of your data talent.
When you have an influx of applicants, it can become overwhelming to screen and interview while trying to act fast and find the best talent.
Transforming data talent might be as challenging as recruitment. Elements can help you quickly zoom into your applicant pool and identify candidates with the exact skills you are looking for so your hiring team does not have to worry about assessing for fundamental technical skills.
You can instead focus on domain knowledge, team fit or go deeper into specific areas. This removes significant workload from your data science teams, who are typically sparse resource for an organization.
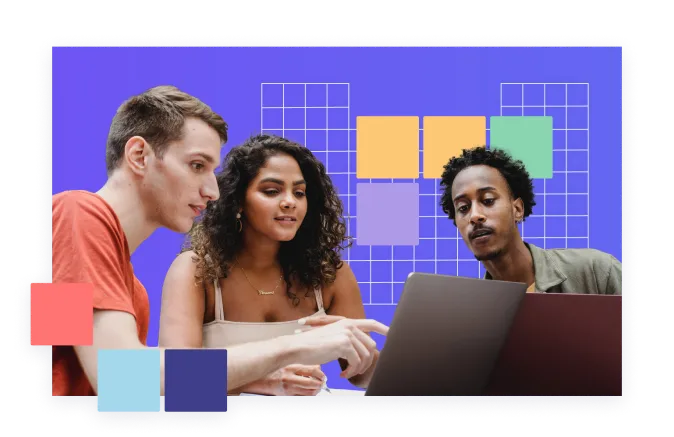
Ready to upgrade your assessment process?
Discover our new data literacy assessments
Created by data scientists and experts
Based in Boston, Elements combines the strong academic research and expertise of
Initiative for Analytics and Data Science Standards with a sharp focus only on data skills.
Each topic is thoroughly covered with highly scaled set of questions created by data scientists and academicians.
Unique assessments from thousands of questions by Elements experts
A different (or the same) set of questions for each candidate
Instant automated performance report for each candidate
Explore Elements
Create. Assess. Track.
Elements is super easy to use, no technical background is required!

Create
- User friendly design for both technical and non-technical users
- Ready to send or highly customizable assessments
- Options to select difficulty and duration
Assess
- Different (or the same) set of questions for each candidate
- Applied coding challenges for Python and SQL
- Optimal candidate experience on a single platform
Track
- Immediate performance reports and scores
- Candidate status tracking
- Analytics to track and optimize the funnel