Descriptive Statistics
Applied Statistics is a critical component of the vast multi-dsiciplinary skills and knowledge set for data science.
A Data Scientist should get their hands dirty with the data; they have to understand and act on all the challenges of real-life datasets.
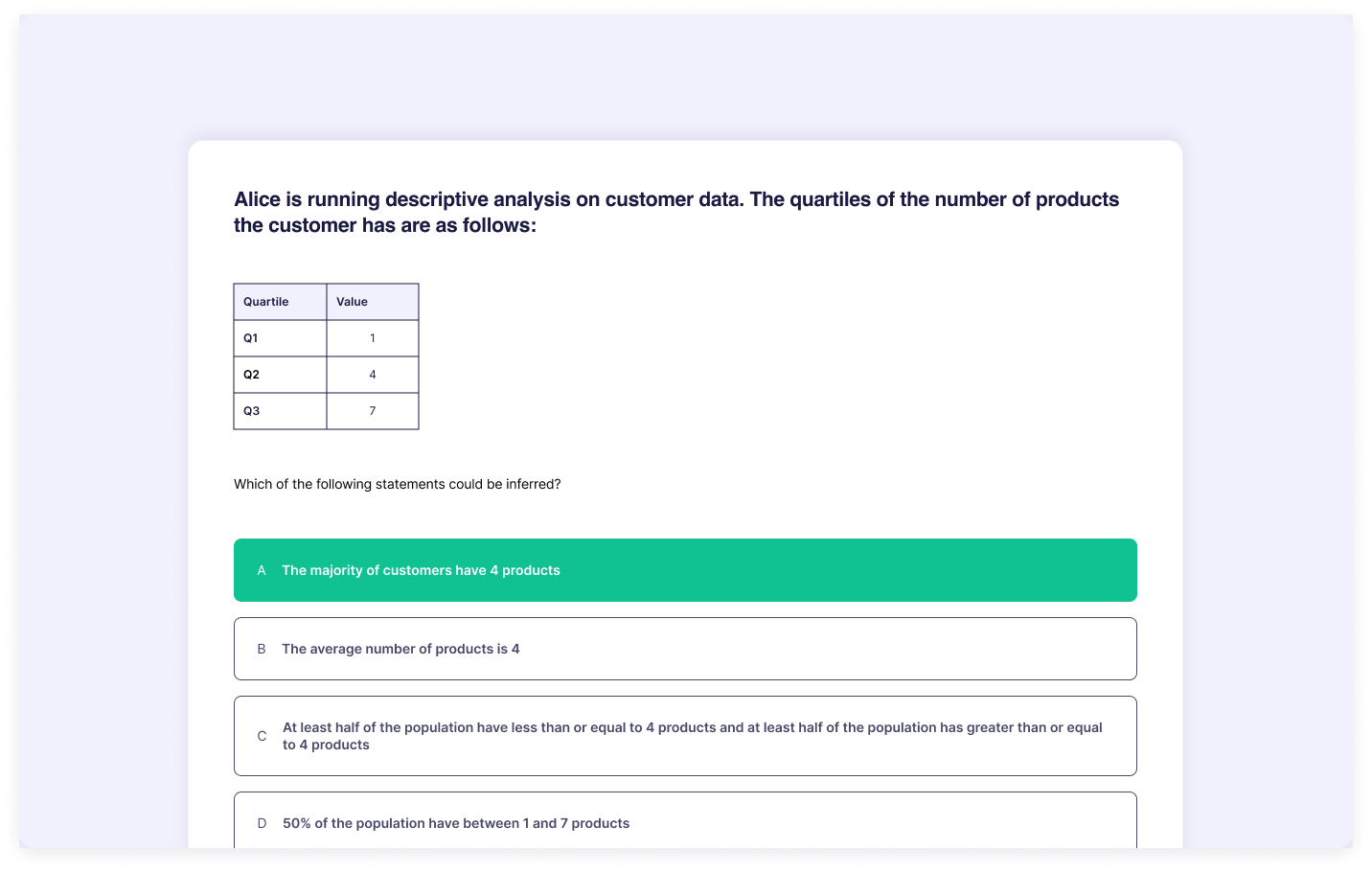
Applied Statistics and its cousin Data Science, though a lot of theory lies behind them, are very practical disciplines. A Data Scientist should get his/her hands dirty with the data; he/she has to understand and act on all the peculiarities of real-life datasets. Though, at least in textbooks, life is presented as usually linear, normal, outlier-free, missing value free, and continuous; the random variables in real-life datasets are far from having these characteristics; they have heavy tails, are multi-modal, have many missing/incorrect values, consist of categorical and ordinal variables with high cardinality, have nonlinear associations between variables, etc. Hence, beautiful theory meets ugly reality. Therefore, a Data Scientist must be equipped to recognize, and deal with these deviations from common 9 assumptions.
A good grasp of Descriptive Statistics has implications in preparing data in the right way for the subsequent inferential statistics phase by using the right transformations and algorithms to extract patterns and to communicate the findings of the data analysis in the right way.
Sample Topics
- Measures of location and central tendency
- Measures of variation
- Measures of association
- Contingency tables
- Visualization of univariate and bivariate data
